What is the future of deep learning? Emerging Applications and Trends
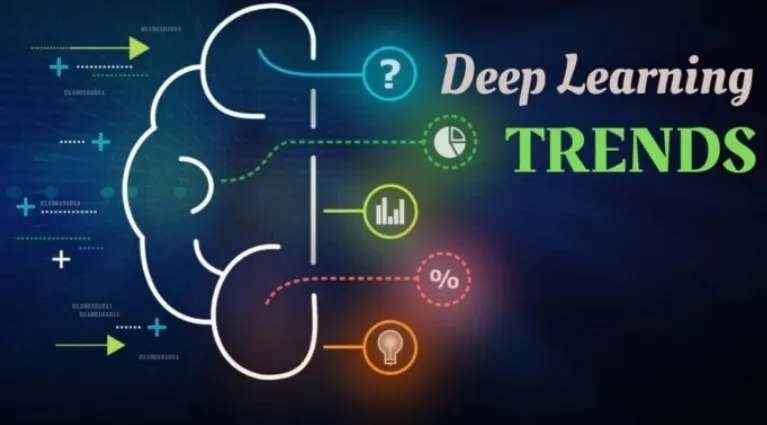
In artificial intelligence (AI), deep learning stands at the forefront, driving remarkable advancements and pushing the boundaries of what machines can achieve. As we gaze into the future of deep learning, an exciting landscape of emerging applications and trends unfolds before us. From revolutionizing healthcare diagnostics to powering autonomous vehicles and enhancing natural language processing, deep learning is poised to reshape industries and transform how we interact with technology. In this blog, we embark on a journey to explore the captivating future of deep learning, unraveling the latest applications, breakthroughs, and trends that promise to shape the AI landscape in the years to come. Join us as we delve into this transformative technology’s boundless possibilities and potential.
Deep learning is experiencing rapid growth, driving transformative advancements across various industries. A deep learning course equips individuals with the required knowledge and skills to navigate this dynamic landscape. Students gain proficiency in developing and deploying cutting-edge deep learning models through hands-on training in neural networks, machine learning algorithms, and deep learning frameworks. Additionally, they learn to analyze complex data sets, optimize model performance, and apply deep learning techniques to solve real-world problems. By mastering deep learning fundamentals, individuals can unlock opportunities in fields such as healthcare, finance, autonomous systems, and natural language processing, driving innovation and shaping the future of AI.
What is deep learning?
Deep learning is a subdo,ain of machine learning that simulates the workings of the human brain’s neural networks in processing vast amounts of data and extract meaningful patterns. It typically involves using artificial neural networks with multiple layers (hence “deep”) to learn and make decisions from complex and unstructured data automatically. Deep learning algorithms leverage hierarchical representations of data to perform tasks like speech and image recognition, natural language processing, and autonomous decision-making. Unlike traditional machine learning approaches, deep learning models can learn directly from raw data without feature engineering. This flexibility and scalability make deep learning a powerful tool for solving complex problems and driving innovations across various domains.
Popular deep learning trends
Explainable AI (XAI): Explainable AI (XAI) refers to the set of techniques and methodologies to provide transparency and interpretability to artificial intelligence models, particularly deep learning models. Understanding their decision-making processes becomes crucial for ensuring trust, accountability, and regulatory compliance as deep learning models become increasingly complex and opaque. XAI techniques enable stakeholders to interpret and explain the reasoning behind AI predictions or classifications, facilitating human understanding and validation of model behavior. Examples of XAI techniques include attention mechanisms, feature importance analysis, and model-agnostic interpretability methods such as LIME (Local Interpretable Model-agnostic Explanations) and SHAP (SHapley Additive exPlanations).
Self-Supervised Learning: Self-supervised learning is a deep learning paradigm where models learn to represent and extract meaningful features from unlabeled data without requiring explicit supervision. Instead of relying on annotated datasets, self-supervised learning algorithms generate surrogate tasks or objectives from raw data and use them to train neural networks. By learning to predict missing or corrupted data patches, temporal or spatial context, or other relevant properties of the data, self-supervised learning enables models to learn rich representations that generalize well to downstream tasks. Self-supervised learning holds promise for addressing data scarcity issues, improving model robustness, and enabling unsupervised or semi-supervised learning in various domains.
Transfer Learning: Transfer learning is a deep learning technique where knowledge learned from one task or domain is transferred and fine-tuned to improve performance on a related task or domain. Instead of training models from scratch, transfer learning leverages pre-trained deep learning models, such as convolutional neural networks (CNNs) or recurrent neural networks (RNNs), which have been trained on large-scale datasets like ImageNet or Wikipedia. By transferring knowledge from these pre-trained models and adapting them to new tasks with smaller datasets, transfer learning accelerates model training, improves generalization, and reduces the need for annotated data. Transfer learning has applications in computer vision, natural language processing, and other domains where labeled data is limited or expensive to acquire.
Edge AI: Edge AI refers to the deployment of artificial intelligence algorithms and models directly on edge devices or endpoints, such as smartphones, IoT devices, sensors, and edge servers, rather than relying on centralized cloud servers for computation and data processing. By performing inference and processing data locally at the edge, edge AI enables real-time decision-making, reduces latency, conserves bandwidth, enhances privacy and security, and enables autonomous operation in resource-constrained environments. Edge AI has applications in various domains, including autonomous vehicles, healthcare monitoring, industrial automation, smart cities, and consumer electronics, where low-latency, privacy-preserving, and decentralized AI solutions are required.
Quantum Computing: Quantum computing is a revolutionary computing paradigm that harnesses the principles of quantum mechanics to perform computations using quantum bits or qubits, which can exist in multiple states simultaneously. Unlike classical computing, where bits represent either 0 or 1, qubits can represent both 0 and 1 simultaneously, enabling quantum computers to perform massive parallel computations and solve complex problems exponentially faster than classical computers. Quantum computing has the potential to revolutionize deep learning and AI by accelerating optimization, sampling, and inference tasks, solving currently intractable problems in areas such as drug discovery, materials science, cryptography, and machine learning model training. Quantum machine learning algorithms, such as quantum neural networks and quantum-inspired optimization algorithms, are being developed to leverage the power of quantum computing to solve AI tasks.
Emerging Applications
Deep learning is catalyzing transformative advancements across diverse domains:
Healthcare: Deep learning is revolutionizing healthcare by bolstering disease diagnosis, drug discovery, and personalized treatment plans. AI-driven diagnostics and telemedicine are emerging as transformative applications, enhancing accessibility and accuracy in healthcare delivery.
Autonomous Vehicles: Deep learning is pivotal in developing autonomous vehicles, powering object detection, path planning, and decision-making systems for self-driving cars. These advancements promise safer and more efficient transportation solutions, heralding a future of enhanced mobility and reduced accidents.
Natural Language Processing (NLP): Deep learning models such as GPT-3 are reshaping language understanding, translation, and text generation. This facilitates more conversational AI assistants and automated content creation, driving efficiency and enhancing communication in various sectors.
Environmental Monitoring: Deep learning aids in environmental monitoring, facilitating climate modeling, wildlife conservation, and disaster response through satellite imagery analysis. These applications contribute to informed decision-making and proactive measures to address environmental challenges.
Finance: Deep learning models are reshaping the finance industry by bolstering fraud detection, algorithmic trading, and risk assessment. Predictive analytics and portfolio optimization leverage deep learning, enhancing decision-making and efficiency in financial markets.
Creative Industries: Deep learning is permeating the creative industries, enabling art generation, music composition, and content creation. Artists and creators leverage deep learning to explore new avenues of creativity, fostering innovation and artistic expression.
Conclusion
The future of deep learning is ripe with transformative applications and trends that promise to revolutionize industries. Pursuing a deep learning course equips individuals with the skills and expertise needed to navigate this evolving landscape and capitalize on emerging opportunities. By mastering deep learning fundamentals and staying abreast of industry trends, individuals can unlock a myriad of career prospects in fields such as healthcare, autonomous vehicles, natural language processing, environmental monitoring, finance, and the creative industries. With deep learning’s pervasive influence across sectors, investing in a deep learning course lays the foundation for a lucrative and rewarding career in the dynamic world of AI.